Machine learning (ML) models depend on the data on which they are trained. Consequently, flaws in datasets can lead to significant vulnerabilities in ML models. ML practitioners frequently tend to focus on “correcting” the model without addressing the quality of the dataset. However, recognizing dataset flaws early can inform and guide subsequent data collection and improve the dataset’s overall quality. In my talk, I will advocate for this data-centric perspective. I will present my research in related areas, including selection bias identification and mitigation, applicability domains, adversarial learning, self-reinforcing bias, and active learning.
18/10/2024 at 13.00
ML Model Reliability From a Dataset Perspective
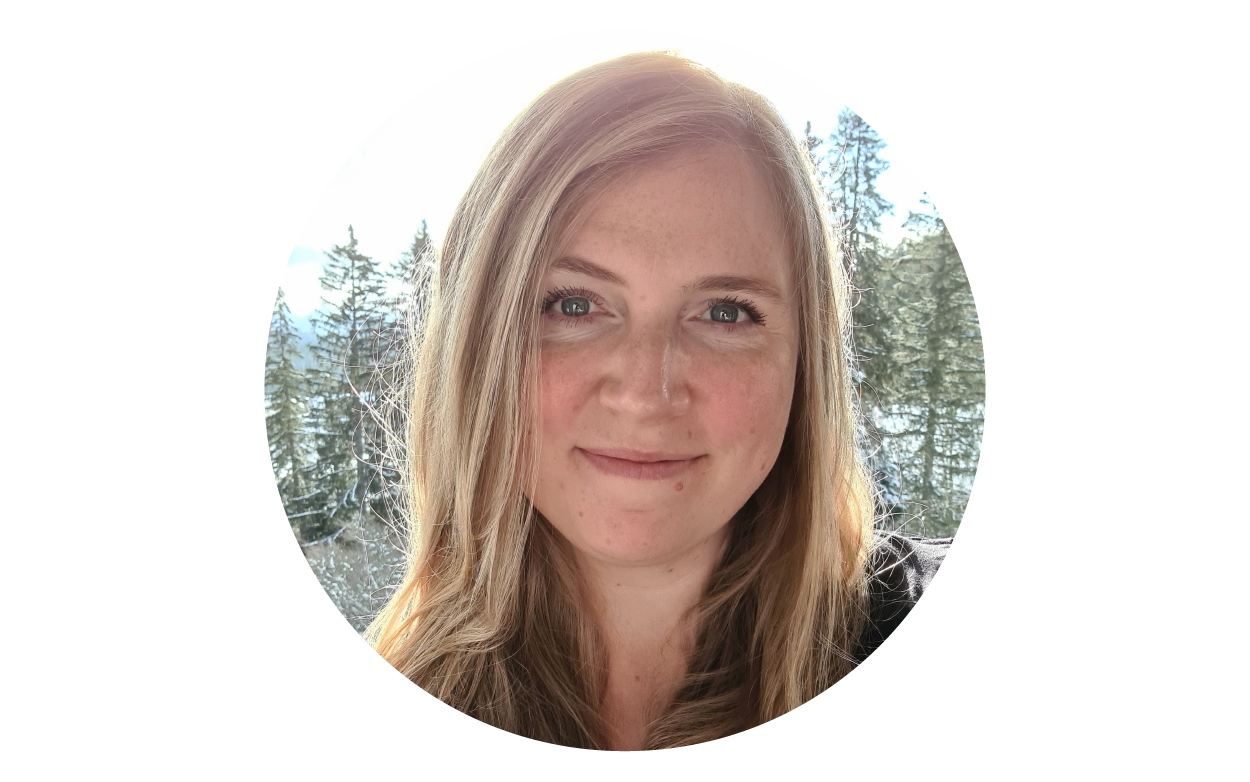
Dr. Katharina Dost
ML Model Reliability From a Dataset Perspective
Katharina is currently transitioning from the University of Auckland to the Jožef Stefan Institute, Ljubljana, Slovenia, where she will continue her work as a SMASH fellow, with a focus on materials science.
Dr. Katharina Dost holds a degree in Mathematics and Computer Science from Johannes Gutenberg University of Mainz, Germany, and a PhD in Computer Science from the University of Auckland, New Zealand. Her main research interests revolve around the reliability of data and models, particularly with respect to biases, adversarial learning, and active learning. She applies her expertise to address challenges in chemistry, healthcare, and environmental science.
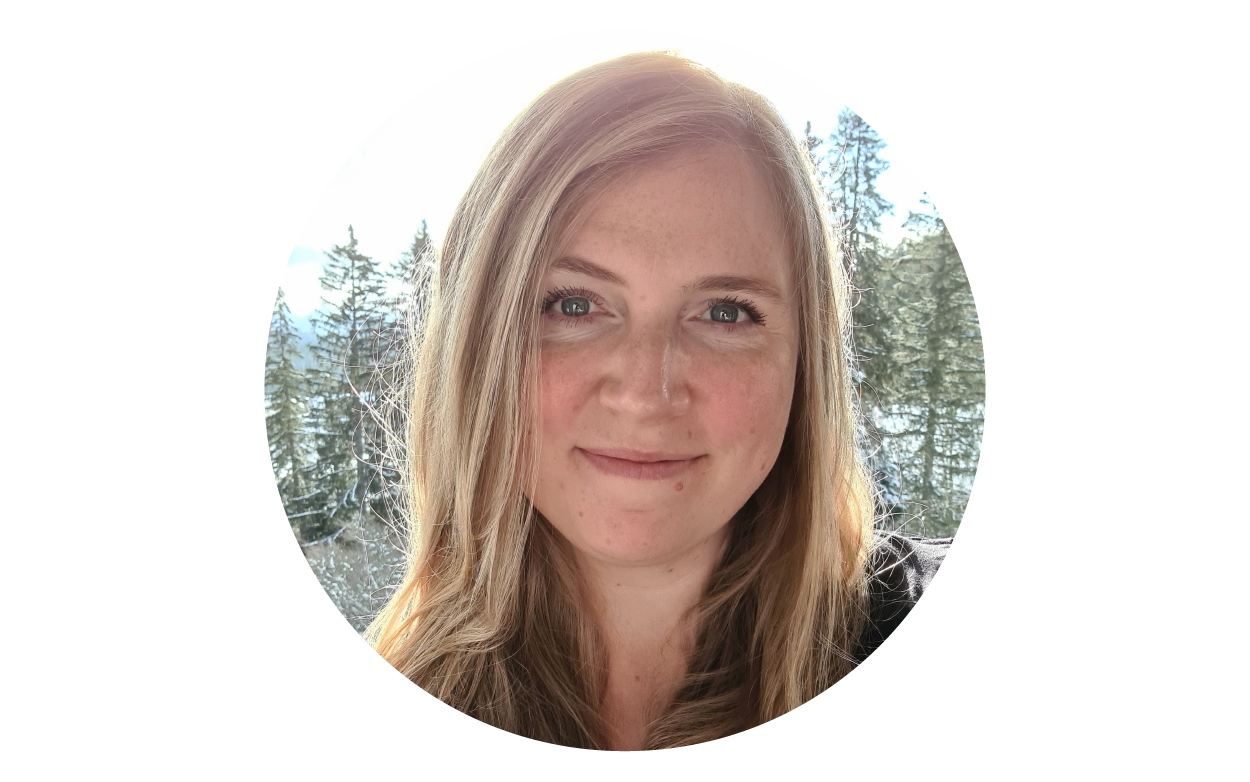
Dr. Katharina Dost
Katharina is currently transitioning from the University of Auckland to the Jožef Stefan Institute, Ljubljana, Slovenia, where she will continue her work as a SMASH fellow, with a focus on materials science.
Dr. Katharina Dost holds a degree in Mathematics and Computer Science from Johannes Gutenberg University of Mainz, Germany, and a PhD in Computer Science from the University of Auckland, New Zealand. Her main research interests revolve around the reliability of data and models, particularly with respect to biases, adversarial learning, and active learning. She applies her expertise to address challenges in chemistry, healthcare, and environmental science.